
Predicting the Unpredictable: How Generative AI is Reimagining Threat Modeling
Predicting the Unpredictable: How Generative AI is Reimagining Threat Modeling

Cybersecurity is a constant arms race, where attackers develop ever-more sophisticated tactics, and defenders scramble to stay ahead. In the ever-shifting chess game of cybersecurity, threat modeling is key—it arms defenders with foresight against attackers' evolving ploys. In the same way a good movie adaptation stays true to the book, effective threat modeling ensures that we're one step ahead of hackers by thoroughly understanding and anticipating their moves. Threat modeling carefully analyzes potential system vulnerabilities so we can build effective safeguards before attacks start. Defending your network is a bit like playing chess; you've got to outthink the hacker, spot the weak spots ahead of time, and have your defenses ready before they make their move.
Traditionally, threat modeling relies heavily on human expertise and established frameworks like STRIDE (Spoofing, Tampering, Repudiation, Information Disclosure, Denial of Service, Elevation of Privilege). Security pros carefully chart out likely threats, weigh their potential damage, and line up defense plans. But let's face it, sticking to old-school manual checks just doesn't cut it against the evolving threats we see today.
Generative AI (GenAI) swoops in to supercharge our threat modeling game, swiftly pinpointing and adjusting to new threats that could slip past even the sharpest security pros. Imagine having a powerful AI tool that can:
- Simulate countless attack scenarios: GenAI models can be trained on vast databases of past attacks and vulnerabilities. Simulating real hacking behavior uncovers vulnerabilities before criminals exploit them.
- Predict future threats: GenAI sifts through threat data, spotting attack trends and weak spots before they hit the mainstream. Staying one step ahead by quickly fixing critical flaws means you're less likely to fall victim to cyber threats.
- Automate tedious tasks: GenAI steps in to take over the grind of sorting through data and spotting security threats, which lets analysts zero in on big-picture tactics and how best to dodge those risks.
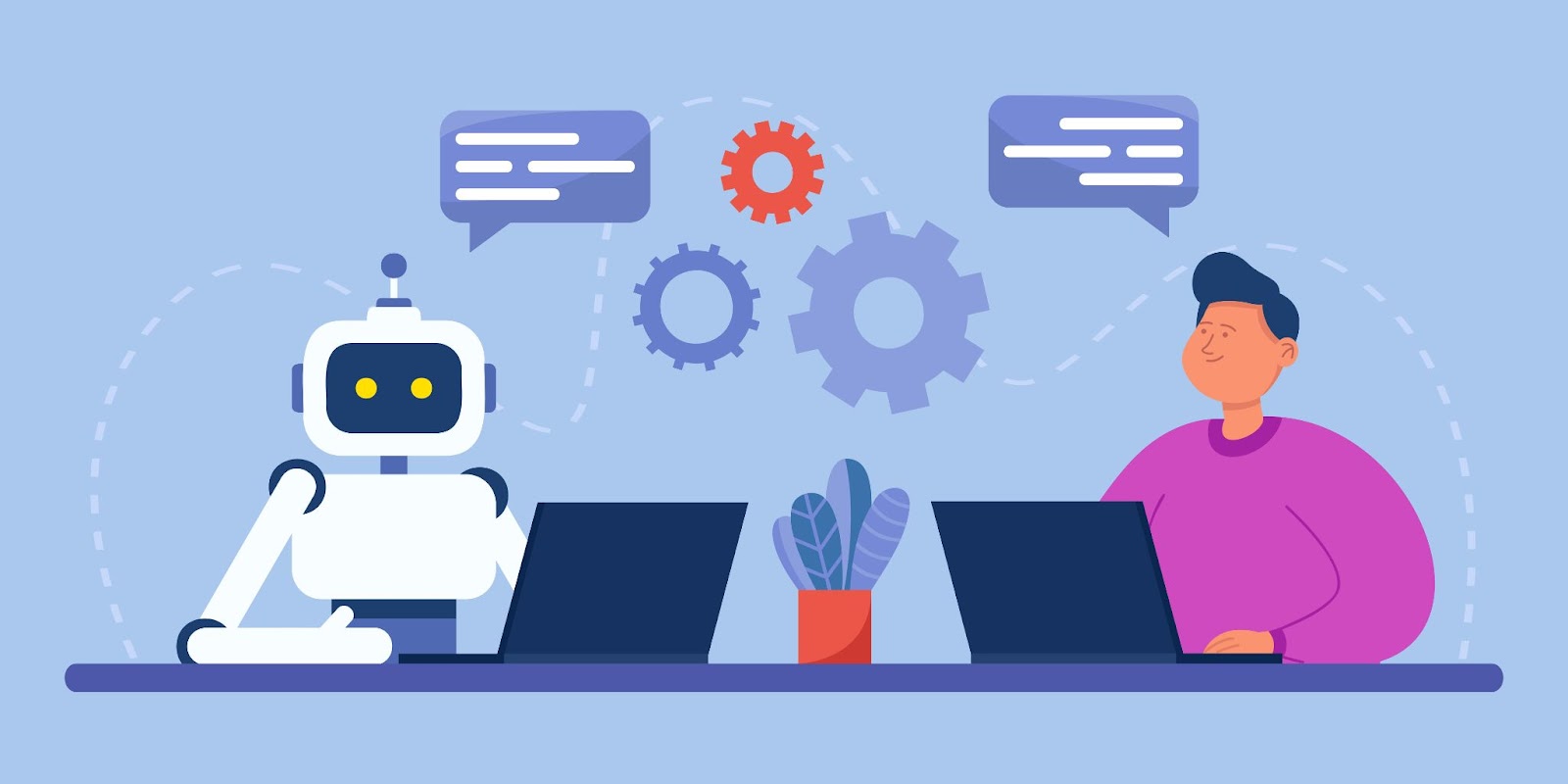
Image by pch.vector on Freepik
GenAI's challenges can be as complex and multifaceted as the world's readers conjure up in their minds from stories. GenAI also comes with its own set of challenges:
- Data bias: GenAI models are only as good as the data they are trained on. Biased or incomplete data can lead to flawed threat models and missed vulnerabilities.
- Explainability and trust: GenAI models can be complex black boxes, making it difficult to understand how they arrive at their conclusions. When GenAI models don't show their work, it's tough to fully trust what they're telling us.
- The human factor: Ultimately, GenAI is a tool, not a replacement for human expertise. Even with AI dishing out smart insights, security pros still have to crack the code and make sharp choices using their smarts and street cred.
How can you smartly tap into GenAI for threat modeling that hits the mark? Here are some key tips:
- Select the right algorithms and datasets: Choose GenAI models specifically designed for threat modeling and trained on relevant cybersecurity data.
- Prioritize data quality and diversity: Ensure your training data is accurate, unbiased, and reflects the latest attack trends.
- Focus on model interpretability: Choose algorithms and training techniques that allow you to understand how the model arrives at its predictions.
- Continuously monitor and refine your model: Regularly evaluate your GenAI model's performance and update it with new data to maintain accuracy and effectiveness.
Picture hospitals, banks, and vital services tapping into smarter AI to stop hackers in their tracks before they wreak havoc. But let's be clear: GenAI packs a punch but it isn't a silver bullet.
Be sure to check out our Must Learn AI Security blog for a deeper dive into the AI threat landscape!
